This article aims to provide an overview of compute governance and the framework designed by Yonadav Shavit (2023) [1]. The authors are not experts in the topic and do not claim to be. Reading into the topic and writing this blogpost was done within 7 hours.
An introduction to compute governance
The fast-paced development of Machine Learning (ML) systems has been an impressive feat to witness during the past few years. In the figure below, you can see the timeline for various deployed models as a function of the number of floating point operations (FLOP) required to train them. With increasing compute and other improvements, state-of-the-art models have achieved impressive capabilities that can be employed in a variety of tasks and environments. The practical uses of these models are increasing and it is becoming more and more pressing to develop regulations and measures regarding their deployment and their use.
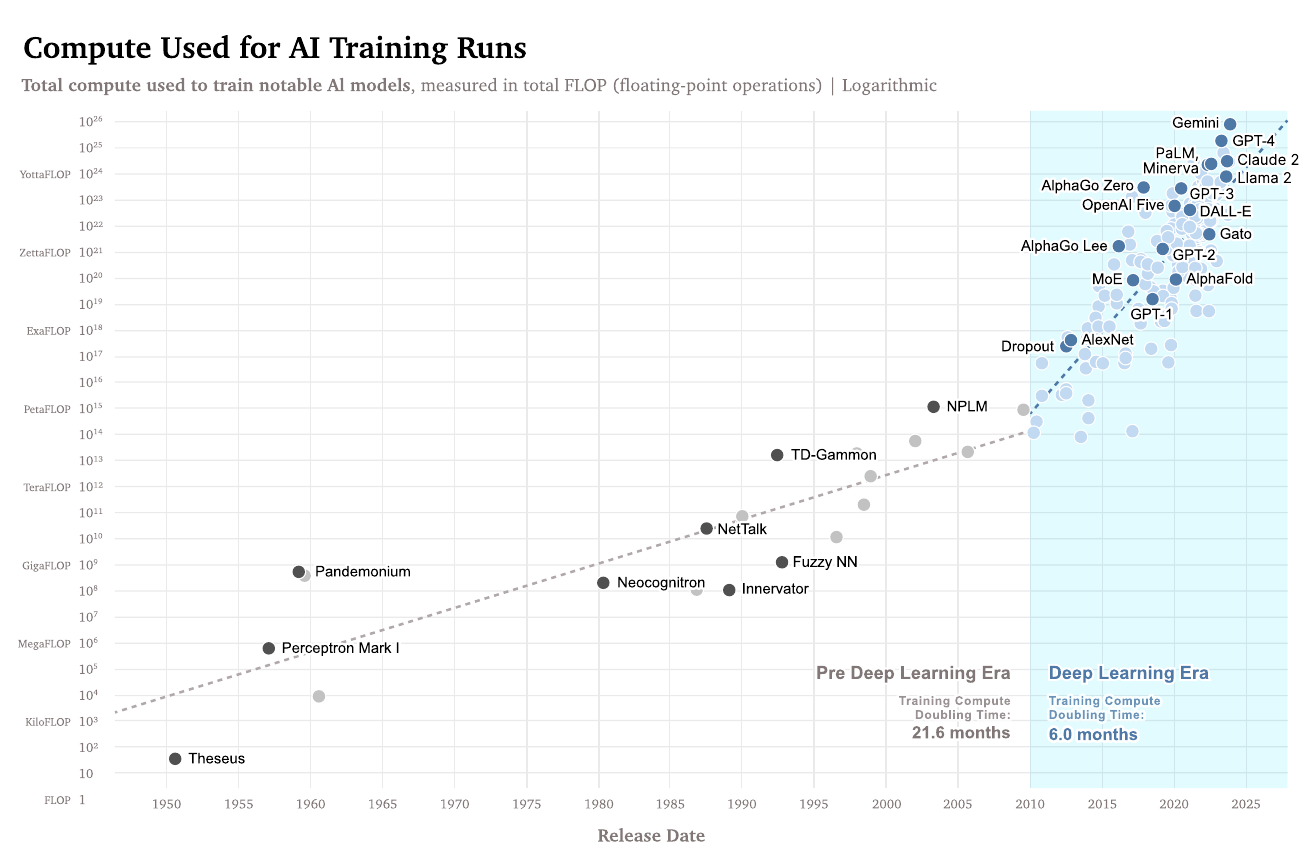
Fig 1: The importance of compute AI in a historical context. [3]
The nascent field of AI governance is tackling this task. In this article, we aim to give an overview of AI compute governance in particular. Recent legislative initiatives have begun to address this area, notably the CHIPS and Science Act in the United States, which aims to bolster domestic semiconductor manufacturing and research and counter China. Additionally, both the European Union and United States have proposed AI acts that incorporate specific computational thresholds for data centres, measured in FLOP. These legislative measures represent initial attempts to quantify and regulate the computational resources utilised in AI development, reflecting the growing recognition of compute as a key factor in AI capabilities and potential risks.
Compute governance can be defined as the control and management of access to compute resources. It stands out as a very impactful approach to governance with a big potential for proposing concrete measures due to the nature of compute hardware [3]:
- Detectability: Compute hardware is highly detectable and governable because of its tangible nature and the substantial resources it requires. This physical presence makes it easier to monitor and regulate compared to digital goods.
- Excludability: Access to hardware is inherently controllable, as possession or rental is necessary for AI model training.
- Quantifiability: Key metrics such as FLOP, chip quantity and quality, computational performance, bandwidth, and memory capacity allow for precise governance measures.
- Supply Chain Concentration: The production of advanced AI chips is highly centralised, with companies like TSMC, Nvidia, and ASML dominating various aspects of the supply chain. This concentration presents both challenges and opportunities for governance.
The Chinchilla framework: A novel approach to AI regulation
In 2023, Yonadav Shavit proposed the Chinchilla framework, aimed at enabling governments or international organisations to monitor and verify AI companies' compliance with regulations while preserving the privacy of sophisticated ML models and avoiding interference with consumer computing devices.
Key components of the framework [1]
This framework has the goal to allow a verifier (e.g. a government) to check if a prover (e.g. an AI lab) complies with the existing legislation on AI safety. It (see figure below) includes three main steps:
- Training data logging: Each chip involved in ML training logs specific traces (weights of the neural network), creating a fingerprint of the neural network training process. These logs are then sent to long-term storage.
- Model parameter storage: Complete model parameters, including hyperparameters and weights, are stored for entire data centres.
- Data encryption and verification: All stored information is encrypted and sent to a verifier, with both the prover (training entity) and verifier retaining copies for cross-checking.
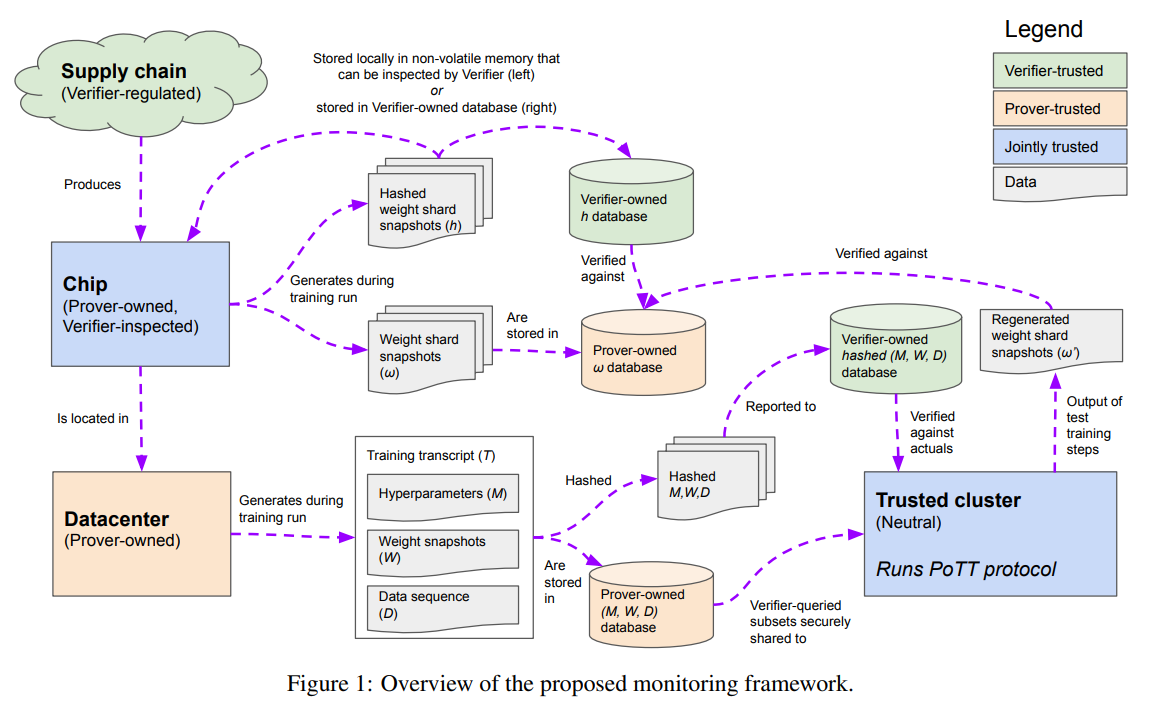
Fig 2: Overview of the proposed monitoring framework [1]
To ensure the integrity of the verification process, the framework introduces a "trusted cluster" – a set of chips that both the prover and the verifier trust. This cluster is designed to protect the prover's data from leaking while performing minimal ML inference and training required for the verification protocol. Regular controls on random samples of chips ensure ongoing compliance. Additionally, supply chain verification is implemented to track ML chips owned by each prover.
Limitations and challenges
While the Chinchilla framework offers a comprehensive approach to monitoring ML training, it faces several challenges:
- Focuses on Large-Scale Data Center Training only: The framework assumes that small-scale ML training does not pose substantial societal harm, an assumption that might overlook potential risks.
- Doesn’t address Existing Chips: The framework does not include any mechanism to control existing chips owned by AI labs.
- Distinguishing ML from Other High-Performance Computing: The framework struggles to differentiate between ML and other high-performance computing workloads on chips. More research is needed on this topic.
On-Chip Mechanisms: Enhancing Compute Governance [5]
On-chip mechanisms represent a promising and necessary approach to enforcing requirements and monitoring the use of compute. These built-in features offer several advantages for regulating AI development and usage.
Key Features of On-Chip Mechanisms
On-chip mechanisms offer robust verification capabilities and product licensing features, enhancing the efficacy of compute governance. These embedded systems can validate claims made by the chip or its owner, enabling the verification of quantitative computations and datasets. This functionality ensures transparency and accountability in chip activities, providing a reliable means of oversight. Additionally, the implementation of product licensing, akin to software licenses, allows for precise control over chip usage based on compliance with regulatory requirements. This feature grants the potential to disable or limit chip functionality if regulations are not met, proving particularly useful in addressing issues such as smuggled chips or non-compliant owners.
Existing Implementations
Interestingly, many of the functionalities required are already in use across various technologies:
- iPhones: Prevent installation of unauthorised applications
- Google data centres: Remotely verify chip integrity
- Multiplayer video games: Use Trusted Platform Modules (TPMs) to prevent cheating
Challenges in Compute Governance for AI [6]
Uncertainty in Computing Performance Trajectories
One fundamental challenge facing compute governance is the uncertainty surrounding future computing performance. While Moore's Law has historically driven exponential growth in computing power, enabling AI advancements through increased training compute scaling, this may not remain to be the case. If Moore's Law reaches its limits, advances in AI may start relying on different innovations, for example in algorithmic advancements, rather than just increasing compute. This would mean that compute is no longer the main driver of AI progress and therefore would reduce the importance of compute governance. Additionally, if the rate of chip developments slows down, so would the frequency of AI chip replacements, reducing the reach of existing governance strategies that monitor chip supply or implement new mechanisms on the latest hardware iterations.
Identifying Regulatory Targets Amid Evolving AI Hardware
As AI hardware continues to evolve, regulators face the complex task of identifying appropriate targets for oversight. The potential use of gaming GPUs for AI computational tasks traditionally reserved for specialised chips raises questions about which types of compute hardware should be subject to regulatory oversight, as indiscriminate targeting of all compute hardware could lead to privacy concerns and overly broad regulation.
Cloud Compute Monitoring
It will be essential to monitor access to cloud compute as more people access compute in this manner. This may even be better for regulation and control as there is potential for more precise controls, control of the quantity actors can access, and flexibility to suspend access at any time.
Challenges in Compute Production and International Cooperation
As more countries develop their own semiconductor supply chains, international cooperation becomes imperative to establish cohesive regulatory frameworks that ensure global standards for security and ethical use of AI technologies.
Limits and Future Directions in Compute’s Contribution to AI
Domain-specific AI applications may achieve dangerous capabilities in narrow fields with significantly reduced compute resources compared to general models. For general-purpose systems, compute serves as a fairly accurate proxy for capabilities, but specialised systems trained for potentially hazardous tasks could be achieved with far less compute. This shift challenges traditional metrics for evaluating compute requirements and calls for adaptive indices that align with specific application domains.
Furthermore, increased compute efficiency and hardware improvements could potentially enable hazardous systems to be trained on a wider range of devices, including consumer-grade hardware; and systems may become trainable on less powerful devices due to increased algorithmic efficiency. This may mean that compute loses its leverage as a leading approach for governance.
Conclusion
In conclusion, compute governance emerges as a critical component of AI regulation, leveraging the unique properties of hardware—detectability, excludability, and quantifiability—to effectively steer AI development. The concentrated nature of the compute supply chain further emphasizes its strategic importance. While frameworks like Yonadav Shavit's proposal offer promising approaches for monitoring and verifying AI development, they also highlight the need for continuous adaptation to technological advancements. The multifaceted challenges in this field, including uncertainties in computing performance, evolving hardware paradigms, and digital access to compute, necessitate proactive, adaptive, and collaborative regulatory strategies. By addressing these issues through international cooperation, stakeholders can foster an environment that promotes responsible innovation while ensuring AI safety.
Bibliography:
- Y. Shavit, "What does it take to catch a Chinchilla? Verifying Rules on Large-Scale Neural Network Training via Compute Monitoring," arXiv:2303.11341, Mar. 2023.
- Konstantin Pilz, & Lennart Heim. “Compute at Scale: A Broad Investigation into the Data Center Industry.”, 2023.
- G. Sastry et al., "Computing Power and the Governance of Artificial Intelligence," arXiv:2402.08797, 2024.
- I. Stevens, "Regulating AI: The limits of FLOPS as a metric," Medium, May 1, 2024. [Online]. Available: medium.com/@ingridwickstevens/regulating-ai-the-limits-of-flops-as-a-metric-41e3b12d5d0c
- O. Aarne, T. Fist, and C. Withers, "Secure, governable chips: Using On-Chip mechanisms to manage national security risks from AI & advanced computing," Center for a New American Security, Washington, DC, USA, Tech. Rep., 2024. [Online]. Available: https://s3.us-east-1.amazonaws.com/files.cnas.org/documents/CNAS-Report-Tech-Secure-Chips-Jan-24-finalb.pdf
- L. Heim, "Crucial considerations for compute governance," Blog - Lennart Heim, Feb. 25, 2024. [Online]. Available: https://blog.heim.xyz/crucial-considerations-for-compute-governance/
This blog post was written with the financial support of Erasmus+
